Data Collection Process
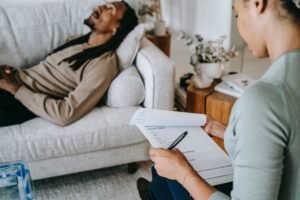
Before embarking on data analysis, either quantitative or qualitative, a researcher is required to collect data. Unfortunately, this is a process that is often underestimated and given little emphasis despite its immense importance. The quality of your data determines levels of reliability, validity and accuracy of the findings. Most researchers fail to involve consultants at this stage ending up with half baked results. For academic research, hiring a dissertation data analysis services will go a long way in having your methodology refined.
The title 21st century will go in history as the century that was characterized by a spike in technology as compared to its predecessors and one that has almost completely shifted all its operations to online platforms. The concept of making the world a global village has relatively been achieved where we achieve miles from the comfort of our seats. However, the digital age has been characterized by data mining and collection for practically every aspect of living. Governments conduct national census and statistics to run the country while companies require your details for you to sign up and with this, they are able to get more information on your lifestyle to strategically place their products and use the same data to learn what the market wants. Learning institutions are no strangers to these systems as they have thrived on them from the early days and this is all that encompasses most of humanity’s learning.
Today we shall be getting to understand this on a deeper and friendly way. NO jargons, but only plain facts in the simplest and most understandable phrasing possible. So, what is data collection? And why is it essential? What are data collection tools and what actors should you consider when choosing data collection tool.
Data collection is the process of gathering information as guided by a laid systematic order in an attempt to test theories, hypothesis or even to answer research questions. Data collection can be as vast as stipulated and could also be restricted to certain scope by given guidelines.
Research goal
I do hold the fact that the best way to work is when you know what your target is and you work towards it. The end game can be stipulated by facts surrounding your research or a targeted result. When looking to introduce a new product into the market, a firm may need a set of data to shine the light on the kind of market they will be meeting. This calls for research on the products in the market by competitors, the age of the target market and their reaction to variables such as pricing and product delivery.
The goal here is aimed at marketing but the same company could hold another research before developing a product and try to understand the gap in the market., This will use a different spectrum and the goal will be to help develop a new product that will be the solution that people need.
The research goal is the driving force behind most research programs as it shows that everything else will have to come to a halt as we wait for this data to give the go-ahead on the direction and possibilities open that can be pursued. In the end, think of data as the bridge or a means to an end.
Statistical significance
Statistical significance is as a result of data representation which is directly determined by the means or data collection tool that was chosen. So, first things first, what is statistics? According to Wikipedia Statistics is the discipline that concerns the collection, organization, analysis, interpretation, and presentation of data. From the basic definition, it suggests that it is inclined towards quantitative data. This kind of data is most suitably gotten using specific methods. While all methods are capable of delivering this to you, it will be more effortless on others and will not disappoint.
Statistical significance of the data is also reflected on the purpose for which the data is being collected, that sets the tone for its significance. Accuracy of the data must be on the higher end of the spectrum and data representation must be done in a form easiest for interpretation such as in a tabular form, chart or even graphically.
Sample size
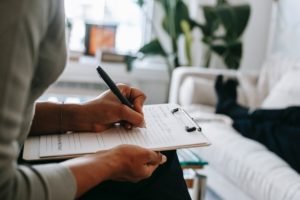
Data collection is not as easy as it sounds as it is the heart of any research. You might have all the technology you need to conduct experiments or theories to test but without data, there is no progress. With this in mind, you need to understand what you are working with. When working with a large group, it might take a long time to get data from each and every unit of the population which is why people opt to go for smaller portions of the whole population which is called the sample. When choosing a sample, there are a couple of criteria that can be used among them simple random sampling, stratified sampling, cluster sampling, systematic and quota sampling among many others. All these have different procedures on how the samples are to be chosen. While one method suggests that the sample be taken randomly in no given order, one will advocate for equality I term of sex, age or even gender.
If you are to work with a large sample then it Is only logical that you use a data collection tool that is both efficient and effective. In this, you would rule out interviewing a large population and instead use questionnaires or another method.
Time
Some research projects are time-sensitive and having the clock ticking on your project is definitely a reason for you to consider all your options. With time being a major factor then you will need to consider what scope you need to cover and what resources you have at hand. With this, you will be in a position to make an estimate of how much time you need to hit your deadline. You will have this as a leading point when deciding on which data collection tool will work for you.
Cost
Money is a strong agent and the world revolves around it. The possibility or impossibility of a task will mostly lie with the availability of resources and money is the first resource to be put into consideration. Give that different data collection tools have different approaches and use different methods to get the required data then this means that the budget allocation of each data collection tool will be different.
This runs right from equipping manpower with all the required resources and facilitating the data collection itself. While some methods are relatively expensive some are very cheap and even considered to be the easiest for anyone on a tight budget. However, at the end of the day, you will have to inject some money in the process.
Accuracy
Different sources of information give a different feel or experience altogether. In this light, they also give different information. Data collection tools may vary in terms of mode of interpretation right to the basic core of the audience involved. In the case of a questionnaire, it is fully dependent on how you phrase the questions and how the target sample interprets the questions. This will make the whole difference. Open-ended and close-ended question receive different results and the limitation to them are quite consequential. ON the other hand, if you opt for a means that will engage you in terms of observation and give you the first hand feeling them this will increase the accuracy of your data.
Depending on why you want this data and what you intend to do with this data then you have to explore different options. In the case of accuracy, it is usually mostly a question of quality which overruns other factors such as cost as it aims at getting the best that is available, think of the national census as the perfect example for this. IT takes time and vast resources to engage in door-to-door interviews for accurate data. This is because the data required is essential to the economic planning of the country and resource allocation. However, this is not a process that is done annually, but the info given will have to suffice for periods of up to a decade.
Validity and reliability
Data validity is a thin line between quality and quantity. Data validity means the presentation of data that serves the exact purpose that it has been extracted for and checks all boxes for a job well done. On the other hand, data reliability means that you can trust the data and use it for the intended purpose without second-guessing it at any point. This is as a result of getting valid data. These two are actually intertwined. Reliable data has to be valid.
The validity and data may take many forms and shapes depending on stipulated conditions. While some sets of data demand to be collected in a certain way, if acquired by any other means it is in violation of basic guiding principles and this invalidates it. Again, I will use the example of a countrywide scenario. During elections, the electoral bodies rely on data collected from all voters to determine who has won in an election. This requires that all voting station be subjected to similar conditions and that all candidates receive similar treatment. Secondly, you cannot take a sample and ask them to vote so as to determine who has won in such an election. When there is a violation of these facts, this leads to a situation of electoral irregularity which can easily render the results null and void and calls for a rerun.
Practicability
So, what does practicability mean in this context? Practicability is basically the unique combination of all the above factors. When considering time, cost, sample size, research goal and validity you end up with the question of how practical are they in relation to the data we need. Time factor will have to be considered but then again how does working under a tight deadline affect the quality of data you need.
The means that how you choose to collect your data should also be tailor-made to suit your target audience. Giving a questionnaire to a semi-literate or illiterate group will render your whole research useless. ON the other hand, if you need to learn of behavioral patterns then you need to have a better feel which calls for observation, videography, surveys or direct participation.
Finally, data security and discretion are also factors to be considered. Some means of data collections lead to incorrect data as they influence the research specimen to act in a different way or shy away from giving accurate inform on or even give no information at all. Silent data collection tools are preferred in such instances as they allow you to get the data needed without the person under review even knowing that you are. This has been made easier in the present day as you can use micro-cameras and recording devise to get that data that you synthesize later. It allows for the most truthful version of the data.
At this point do believe that you are enlightened on what you should consider when choosing data collection tools for your research. Data collection can be done by anyone and it only takes an enlightened mind to decide on the best approach. In case you require assistance, feel free to talk to our dissertation statistics consultant for assistance in choosing and design of data collection tool